Hackathon 0x0d - Slack My Ticket 🧾
Presentation 🤓 In recent years, there has been significant progress in the field of artificial intelligence, especially with the development of lightweight models that can run locally and provide good results. These local AIs are interesting because they ensure data confidentiality, which is a crucial factor in our work environment. As a software company, we use many digital tools to facilitate our work and collaboration among colleagues. Among these tools, we use Slack for internal communication and Redmine for project management.
Read more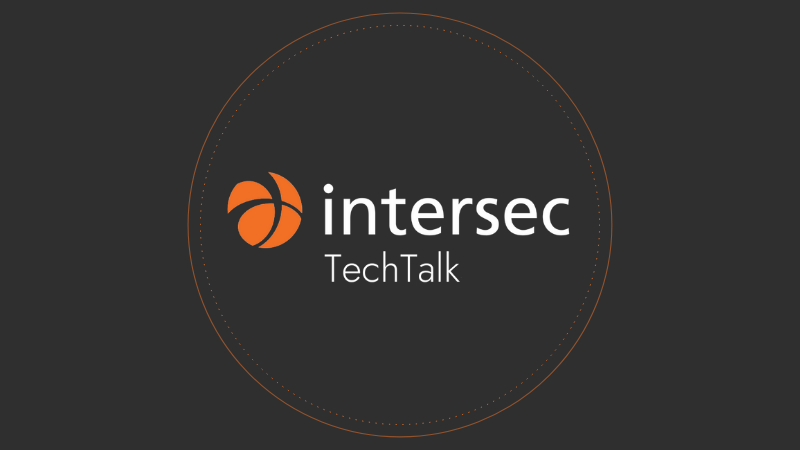